Featured
3 things asset managers should know before using AI as a support tool
Asset managers can utilize artificial intelligence as a support tool. Here’s what to know first before doing so.
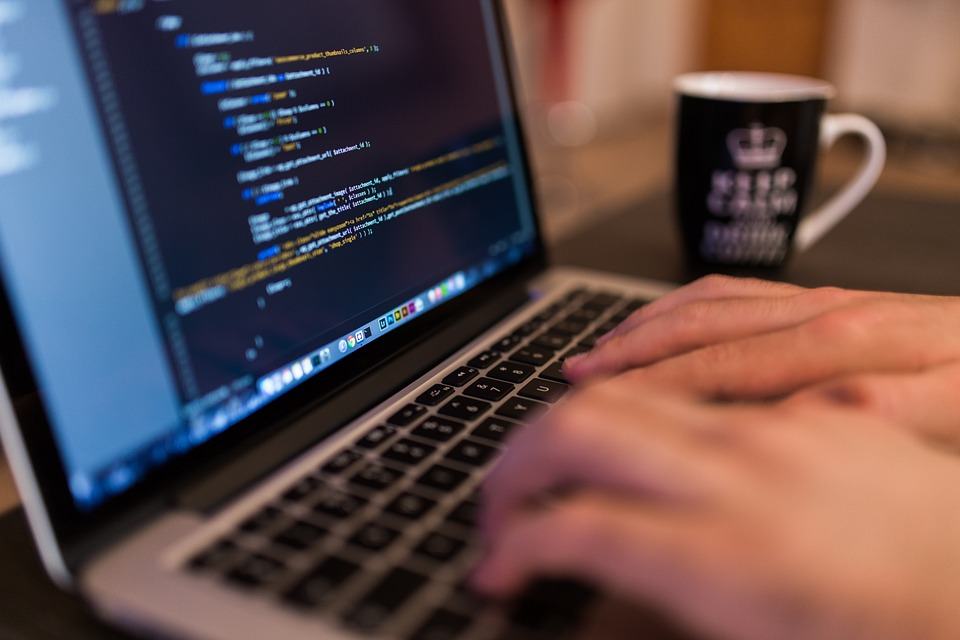
Despite artificial intelligence’s popularity in many industries, its assimilation into asset management is happening at a slower pace. This is a decision-heavy industry in which 74 percent of big decisions are being made by humans, according to a 2017 PricewaterhouseCoopers study. But it’s that over-reliance on the human delegation that makes asset management an industry ripe for disruption.
Early-stage companies across all industries see this shift to AI and are moving toward it in stride. Uninhibited by the old way of operating, these startups will soon allow AI to plug the gaps that current processes used to fill.
Even established powers such as IBM, SAP, Amazon and Salesforce are testing the AI waters. Amazon is already looking to disrupt banking and real estate, and some analysts believe both it and Google could transition into asset management.
The only way to keep up with the rapid pace of technology is to find unique ways AI can support the current business. For asset managers, that means using AI as a complementary tool that helps them provide more well-rounded services to clients.
The reality of AI
When AI first hit the scene, much of the talk surrounding it focused on how robots would inevitably replace human workers. This sentiment is a bit overstated. Yes, many tasks that humans currently perform can be better performed by machines, but humans won’t be replaced — just reassigned.
In the asset management industry, the worry is that people won’t trust financial decisions made by machines. Technology lacks the contextual experience that humans possess, meaning one bad decision could endanger your customers as much as it damages your company. Your computer doesn’t pay rent or utilities to live in your home, so how could it match a human’s gut instinct to account for those responsibilities when managing a portfolio?
Leaders understand the pros and cons of a full-scale AI implementation, but they understand that automated assistants are increasingly useful for tasks in their organizations. A ServiceNow survey found that 92 percent of HR leaders believe that chatbots and conversational interfaces will facilitate intra-company communication in the future. These statistics display that companies in several industries are successfully introducing AI both internally and externally.
Asset managers should see this information as validation that AI won’t swoop in and negate the human element. It can help asset managers take an advisory role online and offline while maintaining the usefulness of in-person sessions.
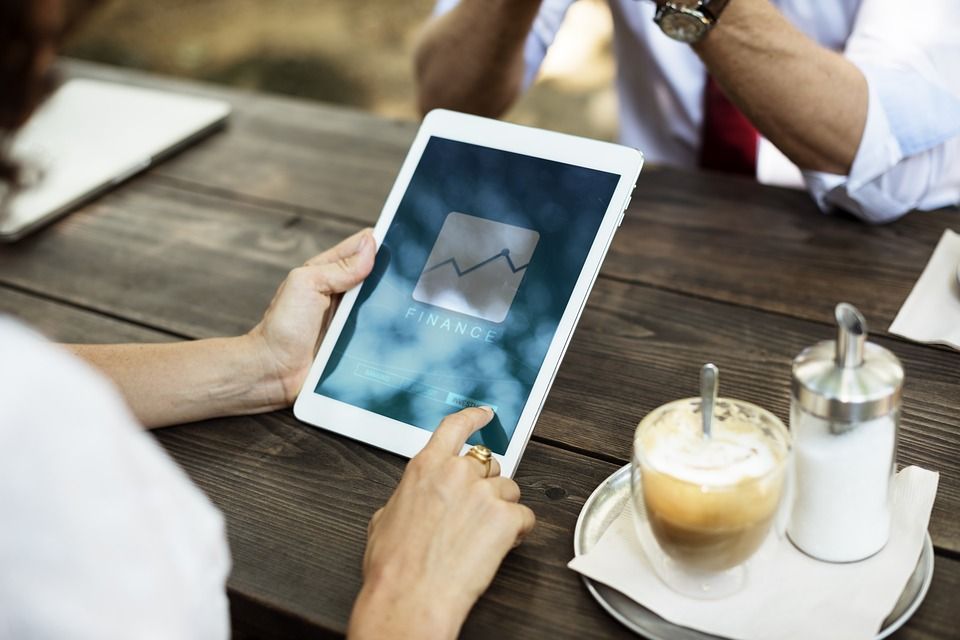
AI could be the next disruptor in the finance sector. (Source)
How to make AI an ally
An investment firm needs to process a large volume of data and information on any given day. While humans have the ability to make great decisions, computerized tools can help support this decision-making further. AI has the capacity to analyze significant amounts of data, faster and with more accuracy than a team of humans can do alone. Firms can successfully implement AI if they adhere to three important strategies:
Let data be the guide.
Goldman Sachs and other investment firms already have either an in-house or consulting data scientist. That’s great, but it’s not AI. The difference is in scalability: Whereas a data scientist is a great asset, he or she can handle only so much volume.
AI needs access to the same data sources a data scientist would. Unleashing an AI bot on the stock or commodities markets, for example, would give the program enough data to learn, find patterns, and adapt to the market. Determining the right data sources and figuring out the time needed to properly train the platform is the first step to implementing AI.
Ensure that the AI strategy is scalable.
Digital disruption moves fast, and new data streams pop up seemingly every day. The real value in AI is its ability to learn and adapt to these changes and make its own correlations without human intervention. If you must continuously retrain the tool and introduce new data models, then it’s no different from a human employee. That’s not a scalable business model. The real innovation in AI comes in when it adapts to new data streams and builds data models in real time.
Due diligence is important for investment firms because not everyone seeking funding is honest and transparent. No two deals are alike: One company’s value may be in its intellectual property, while another’s in the founders. Though you can’t expect AI to understand these key differences, it should be able to present the information for asset managers to make the right decisions. This is where the role of semantic design and context awareness differentiates a unique AI strategy from a mediocre one.
Keep the algorithm focused.
In the current phase of AI advancement in which most algorithmic capabilities lack domain knowledge and context, a proper AI algorithm needs to be laser-focused. For example, if an AI tool is very good at monitoring savings and retirement accounts, it has repeatability. It can continuously monitor data in those markets and eventually be adapted to do more. The more focused the model, the more valuable and scalable it is.
Attempting to build a one-size-fits-all model is a lost cause. While an algorithm built for savings accounts could be useful in creating another for monitoring stocks, they’re different markets that ultimately require different algorithms. Just as you wouldn’t use a screwdriver to hammer a nail, use caution when migrating AI bots into different verticals. When companies advocate AI tools in image recognition and want to apply them in banking, they should consider how much more they will need to pay when their data complexities increase.
AI drives the next disruption in the financial sector. Companies around the world, especially in the tech sector, are already researching ways to implement it. Every industry should look into how this technology can supplement existing processes. The key is to focus more on building decision-support tools versus decision-making tools. Humans, not robots, will still be the key users of AI.
—
DISCLAIMER: This article expresses my own ideas and opinions. Any information I have shared are from sources that I believe to be reliable and accurate. I did not receive any financial compensation for writing this post, nor do I own any shares in any company I’ve mentioned. I encourage any reader to do their own diligent research first before making any investment decisions.

-
Biotech1 week ago
Biotech’s Comeback: AI, M&A, and Innovation Drive New Growth
-
Business2 weeks ago
How Overvalued is the Dow Jones?
-
Crowdfunding5 days ago
FoodSeed 2025: The Third and Final Call for Italian AgriFoodTech Startups Is Underway
-
Crypto2 weeks ago
Crypto Markets Struggle as Inflation and Fed Policy Weigh on Bitcoin
You must be logged in to post a comment Login