Business
Digital transformation: The data science challenge
Due to data explosion and digital transformation, data scientists are a must now for organizations.
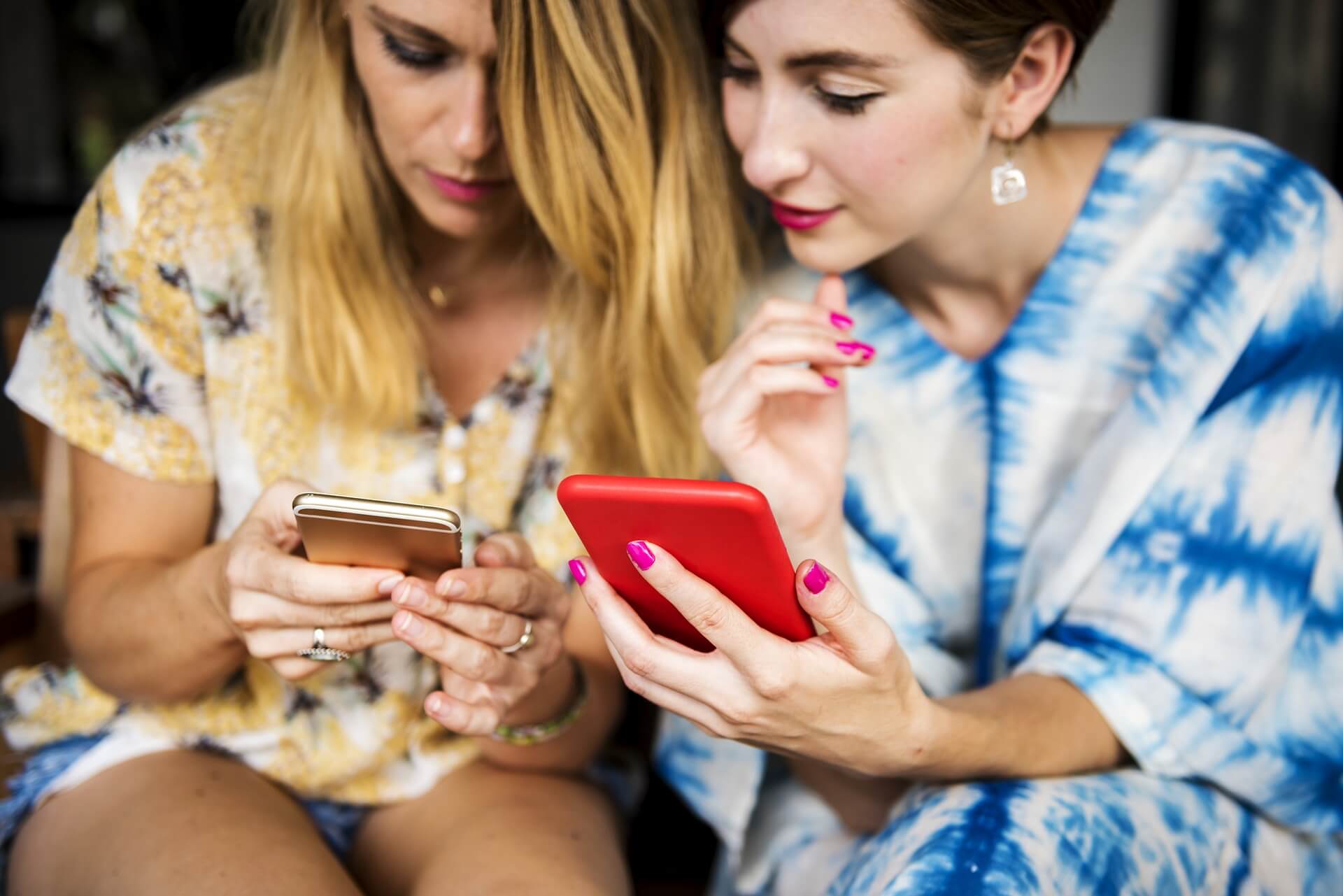
The explosion of data, ranging from social sources like Facebook to sensors in the Internet of Things, has created a need to stem the flood and make sense of what is in there. There is also the promise of new insights gleaned from patterns in the data that will predict scenarios and not just report on what happened in the past.
This has compelled many organizations to hire data scientists to cast light on the mysteries found in NoSQL databases and data lakes where all this data is being accumulated. However, the results have often been rather disappointing. In a white paper on Data Science, Pivotal reports that $41 billion was spent on data science software and infrastructure in 2015, according to research by IDC. This excludes staffing and other costs. However, the ROI for all this expenditure exceeded three percent for only 27 percent of a global survey conducted by Teradata for Forbes Insights, in which they interviewed 316 executives of businesses with a minimum turnover of $500 million.
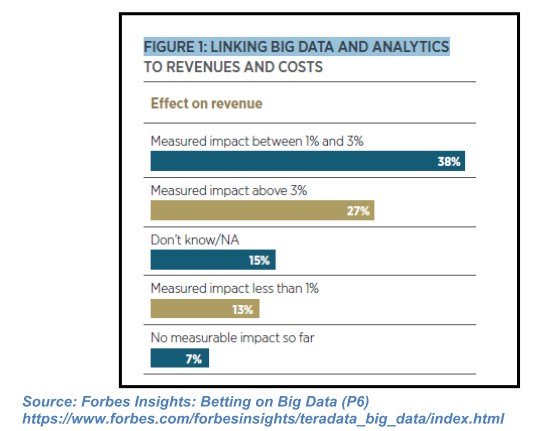
© Georgi Todorov
So why the big discrepancy between expectations and delivery? There are a number of factors contributing to this disappointing state, such as the following:
- Meaningful data access only available to the privileged few.
- A lack of strategy and vision as to what big data should provide to the organization.
- Not recognizing that other role players are required to support and interact with the data scientists in a viable business unit.
- A lack of appropriate business processes to support the big data value chain.
Data for All
Traditionally, business intelligence has been restricted to the IT division, which produced standard reports, using historical data from structured databases and data marts. Ad-hoc requests could take weeks or even months to be delivered. In this era of big data, this is not good enough or fast enough.
Becoming a data-driven organization requires everyone in the company to have an unfettered access to data, limited only by security considerations. Once they have the access, they should be encouraged to use it and familiarize themselves with what is available. This should start as a top-down initiative, with executives showing the way. This is a big cultural change and is probably the hardest part of the data-driven journey. The Forbes survey found cultural issues to be prominent in most companies, even those who were succeeding in their transformation.
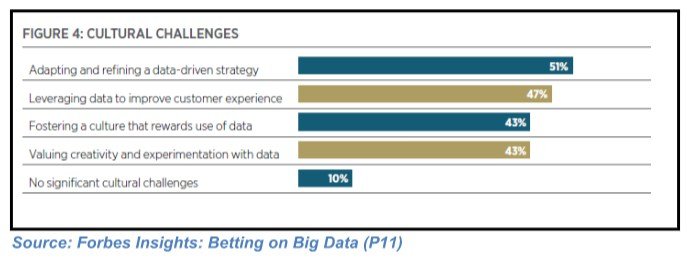
© Georgi Todorov
Creating the Vision
There are many aspects to becoming data-driven, and not the least of them is how to manage the tsunami of data that you will need. Master data management in the age of big data is complex and we discuss it in a separate article. What is more, analyzing data is not an end in itself, since someone needs to apply the analytics if they are to have any value. While there is much buzz about AI and machine learning, in the short- to medium-term this job is still reserved for humans.
You also need to determine where you will apply analysis first; maybe risk management, for example, or demand forecasting. Build an attainable roadmap, engaging as many of your employees as possible, as the start of your change management process, and implement a communication program to report progress to all employees and stakeholders. You will also need to make adjustments to your business model and organizational design if you want to embed data science in your business.
A Data-Driven Business Model
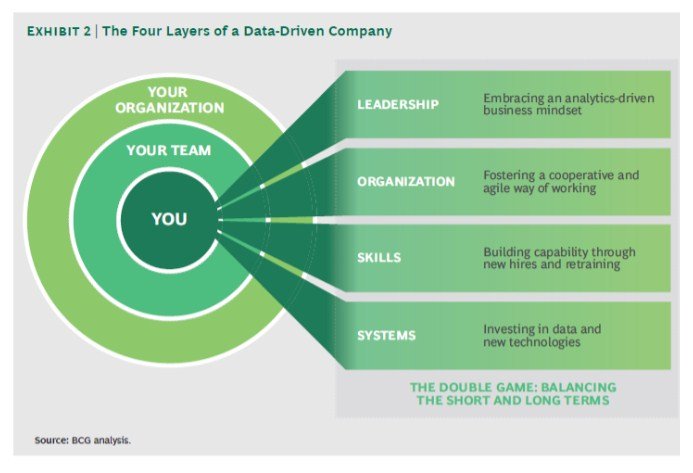
© Georgie Todorov
There are two schools of thought about how you deploy data science and analytics – centralized or decentralized. The centralized one is closest to the traditional business intelligence set-up. Most BI business units are located in IT and report to the CIO. Ideally, you need a CDO (Chief Data Officer) who is on par with your CIO, to whom the data science/analytics team will report. The analytics team should include business users, who will both initiate the business need and be responsible for applying the output.
This is why many companies have a decentralized approach, where data scientists and analysts are deployed to different business units. Obviously, this is viable only for large-scale enterprises who can afford to appoint enough data scientists. You could look at a long-term co-sourcing option, where you retain the services of a company that has the necessary big data experience to perform the analytics. There needs to be a good long-term relationship in this scenario, as the data scientist(s), analyst(s) and your business unit managers will be working together closely.
For example, apart from a core team of employees, AIG Insurance employed over 100 candidates of their centralized “Science Team,” with skills as diverse as psychology and behavioral economics to master their data challenge. They prefer to call the outputs from their Science Team Evidence-based information. Decisions on what to do with the information are strictly human, carried out by industry experts who use their intuition and experience to maximize these insights and predictions.
Implementation and Operationalization
The collection, refinement, and mining of data is the start of the journey to the intelligent use of this data. Apart from an organizational design that supports data analysis, there needs to be a process that ensures that a decision to act on the information takes place. This may sound common-sense, but the diagram below from Pivotal indicates how poorly data is utilized in general. It is also a good indicator of why the ROI obtained is so low generally.
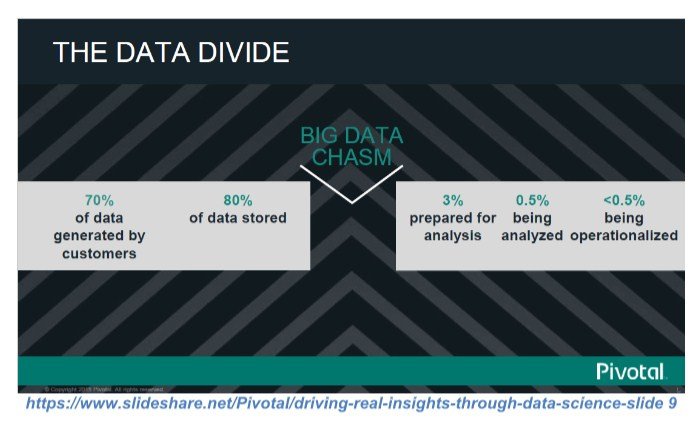
© Georgie Todorov
The amount of data that is analyzed is minimal, and even less of it is operationalized. However, even 1% can make a difference, as illustrated by these results from General Electric in the same slide deck.
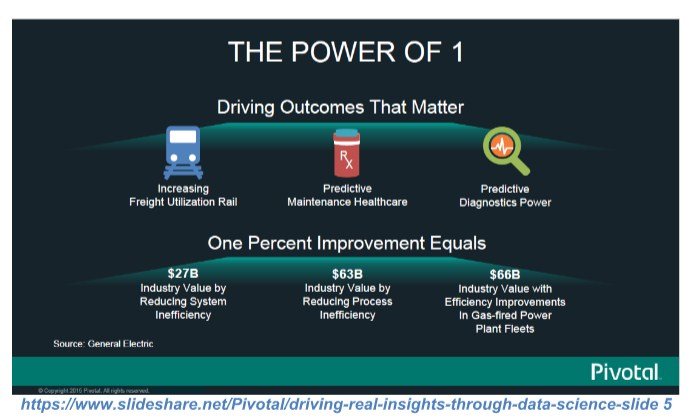
© Georgie Todorov
Pivotal attribute the poor ROI to a number of operational issues, starting with a lack of process integration, where business intelligence is delivered in a disparate system to the user. The latter must try and reconcile what is presented in the BI with the systems that are affected, as cited by a worker who needs to switch back and forth between the BI system and the ERP system to check on some anomaly in inventory management. This would cause the worker to disengage from the process, especially if there has been no change management around the importance of using data. The process should monitor whether analytics are applied or not, and why. It is recommended that a service provider is consulted to assist in building a robust process.
Some other issues around operationalization mentioned by Pivotal include:
Failure to act on “perishable” data
• Perishable data is data that has a very short lifespan—it must either be acted on immediately or discarded. Typically this could be IoT data. If a customer is passing by a beacon in a store, it is no good sending a special offer about an item on the nearest shelf half an hour later.
Timing issues
• The right data must be delivered at the right time, especially where decisions are time-bound, like ordering seasonal inventory such as umbrellas. Also, where data is being combined from different sources, synchronicity is important.
Bismarck said that those who fail to learn from history are doomed to repeat it.
• This is often the case where predictive analytics are used without reference to historic data. Predictive analytics is often insufficient to make relevant business decisions. For instance, recommending that more umbrellas should be ordered does not necessarily give enough information on what quantities should be supplied to a particular store without looking at historic sales.
At the end of the day, the human element is still the most important factor in the transformation. Ernst and Young surveyed over 500 companies globally on their data transformation for Forbes. Leading companies understand that not only must they make their employees “data-conscious,” but they must enable them to act on data insights with as little obstruction as possible.
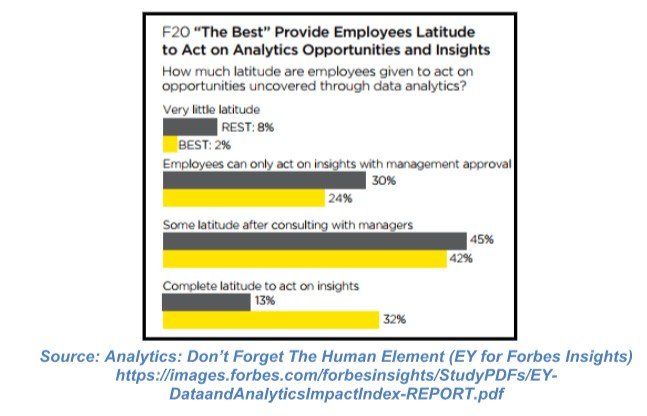
© Georgie Todorov
There is no point in providing data insights if the ability to take action is wrapped in red tape.
Is It Really Worth the Effort?
Well, yes, if you look at the diagram below, from Boston Consulting Group’s article. This year, 6 out of the top 10 largest companies by Market Cap. are data-driven.
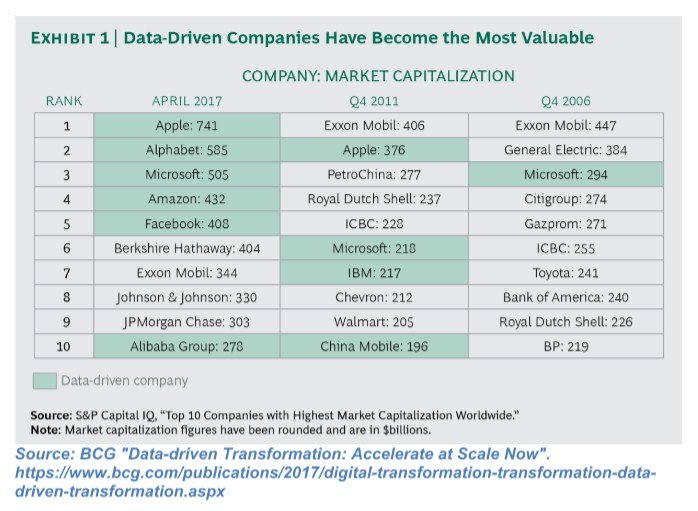
© Georgie Todorov
Alphabet (Google) and Apple have had an ongoing battle for top space, with Apple reigning triumphant for now. What is remarkable is how oil companies and banks are gradually being squeezed out by companies such as Facebook. However, there is no easy transition. If the largest multinationals in every sector, from banking (like Citigroup) to healthcare (like Johnson & Johnson) are struggling to get to grips with in-house data science initiatives, it is an even bigger challenge for smaller companies with correspondingly smaller budgets and less leeway to take risks.
As we have noted above, investing in data scientists and neglecting the changes to your business model and culture can result in a disappointing result. To start the journey with minimal risk, you should define a pilot exercise and find a company who can help you with your initiative. The expected outcomes should be:
- a successful delivery of the pilot output
- a defined process for analytics management from the concept to applying what was delivered from the analysis
- an idea of whether you should in-source, outsource or co-source
- a decision taken on whether you should have a centralized or decentralized approach if you in-source
The transition to a data-driven culture takes some time, but it is part of your route map to true digital transformation. It will also propel you past your competitors, as the graph below indicates.
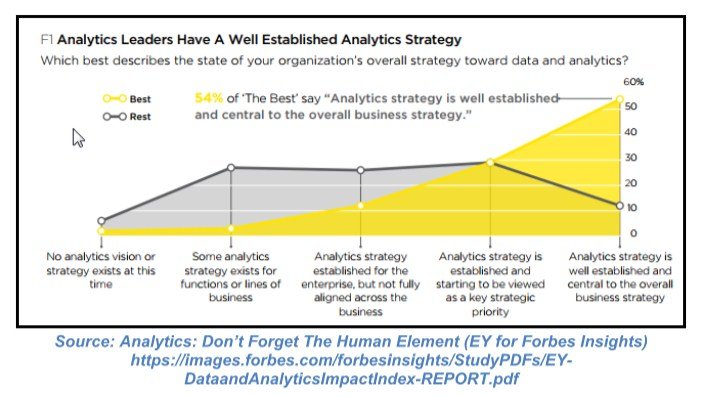
© Georgie Todorov
(Charts courtesy of Forbes Insights, Slideshare, BCG Analysis.)
—
DISCLAIMER: This article expresses my own ideas and opinions. Any information I have shared are from sources that I believe to be reliable and accurate. I did not receive any financial compensation in writing this post, nor do I own any shares in any company I’ve mentioned. I encourage any reader to do their own diligent research first before making any investment decisions.

-
Crowdfunding1 week ago
PMG Empowers Italian SMEs with Performance Marketing and Investor-Friendly Crowdfunding
-
Markets4 days ago
Markets Wobble After Highs as Tariffs Rise and Commodities Soar
-
Markets2 weeks ago
The Big Beautiful Bill: Market Highs Mask Debt and Divergence
-
Africa1 day ago
ORA Technologies Secures $7.5M from Local Investors, Boosting Morocco’s Tech Independence
You must be logged in to post a comment Login