Featured
How are ESG company news deciphered with Artificial Intelligence
Thanks to the incredible progress in artificial intelligence (AI) observed in recent years, and more specifically in natural language processing (NLP), the RAM-AI research team has been able to rely on linguistic algorithms to transform textual data into digital representations, while preserving semantic proximity in a digital space.
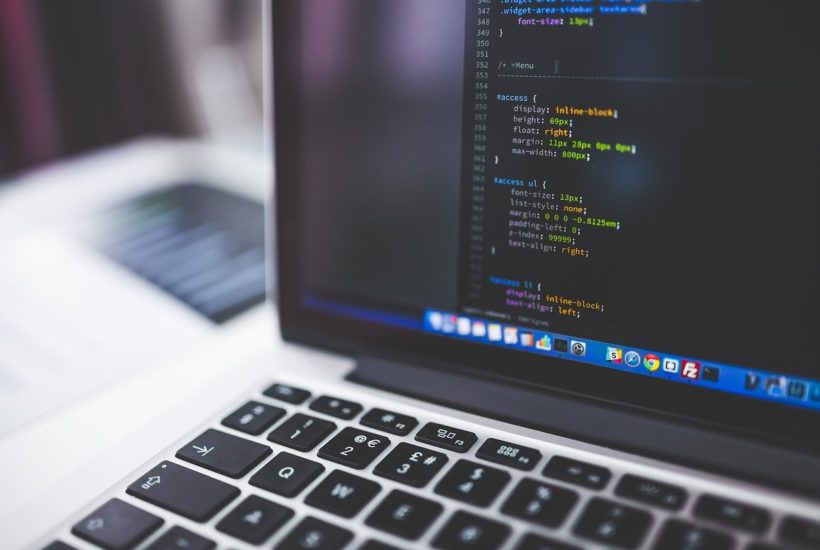
A trend that has already started in recent years, the integration of ESG (Environmental, Social, and Governance) criteria has, since the COVID crisis, become an essential part of business analysis. The evaluation of financial characteristics is complemented by an assessment of the sustainability of the activity, its climate and societal impact, and the resulting investment risks.
In quantitative finance, the recent proliferation of ESG data, reported by third-party analysts or by companies alongside their income statements, allows for systematic incorporation into investment algorithms. Most of this data is so-called “structured”, i.e. presented in numerical form and therefore easily analyzed, modeled, and then integrated into a quantitative process.
Read more about the RAM-AI research on ESG company news and find the latest business headlines with the best online news aggregator, our companion app Born2Invest.
The topics addressed focus mainly on the health and well-being of employees
Financial markets are, however, influenced by a multitude of signals, which can sometimes take less standardized forms. In addition to conventional structured data, it therefore seemed essential to explore the predictive power of so-called “unstructured” information, representing all non-numerical data such as text or images. At the forefront of the RAM-AI research is the systematic analysis of media information flows (press, websites, social networks, …) on ESG topics, for two main reasons.
Firstly, many studies have highlighted the links between a company’s media activity and future movements in its action. Secondly, these dynamic flows are a perfect complement to traditional ESG data characterized by a very low discounting (often annual). This approach is therefore essential in such a volatile market context. The ESG themes captured by algorithms, in the pre-COVID (December 2019) and post-COVID (June 2020) periods focus mainly on employee health and well-being, topics that have only anecdotally appeared before.
The integration of unstructured data brings an additional difficulty: due to the symbolic nature of the text, quantitative models cannot directly assimilate the information. Thanks to the incredible progress in artificial intelligence (AI) observed in recent years, and more specifically in natural language processing (NLP), the RAM-AI research team has been able to rely on linguistic algorithms to transform textual data into digital representations, while preserving semantic proximity in a digital space.
Most of these machine learning models (BERT, RoBERTa, …) have been developed by major technological players (Google, Facebook, …) in “open source”. They were then adapted to meet our needs in a precise way. Indeed, the basic algorithms have been trained on general text corpora such as Wikipedia, and therefore cannot capture all the nuances of information related to the ESG information flow. A “fine-tuning” process based on a large ESG text database was therefore introduced.
An example of the use of RAM-AI infrastructure is the prediction of the volatility of the shares of companies mentioned in media feeds. RAM-AI quantitative approach integrates more than ten years of structured and unstructured data to train neural networks to identify interaction patterns, and thus estimate future volatility. In the graph below (simulations based on the European market), stock volatility is indeed much higher/lower when, following an ESG media flow, the predicted volatility is high/low (Qu_4/Qu_0).
__
(Featured image by Pexels via Pixabay)
DISCLAIMER: This article was written by a third party contributor and does not reflect the opinion of Born2Invest, its management, staff or its associates. Please review our disclaimer for more information.
This article may include forward-looking statements. These forward-looking statements generally are identified by the words “believe,” “project,” “estimate,” “become,” “plan,” “will,” and similar expressions. These forward-looking statements involve known and unknown risks as well as uncertainties, including those discussed in the following cautionary statements and elsewhere in this article and on this site. Although the Company may believe that its expectations are based on reasonable assumptions, the actual results that the Company may achieve may differ materially from any forward-looking statements, which reflect the opinions of the management of the Company only as of the date hereof. Additionally, please make sure to read these important disclosures.
First published in allnews, a third-party contributor translated and adapted the article from the original. In case of discrepancy, the original will prevail.
Although we made reasonable efforts to provide accurate translations, some parts may be incorrect. Born2Invest assumes no responsibility for errors, omissions or ambiguities in the translations provided on this website. Any person or entity relying on translated content does so at their own risk. Born2Invest is not responsible for losses caused by such reliance on the accuracy or reliability of translated information. If you wish to report an error or inaccuracy in the translation, we encourage you to contact us.

-
Crypto4 days ago
Bitcoin Recovers After U.S. Strikes Iran, While Altcoins Face Sharp Losses
-
Business2 weeks ago
Gold Closed the Week at a New All-Time High As the Dow Jones Continues its Pattern of Weakness
-
Africa14 hours ago
Morocco’s Wheat Dependency Persists Despite Improved Harvest
-
Crypto1 week ago
Brazil’s Crypto Boom Threatened by Surprise Tax Proposal