Business
Here’s how predictive analytics can improve equipment maintenance
Predictive analytical models are an important tool for engineers, and they’re also a revolution in product and parts testing and even business forecasting.
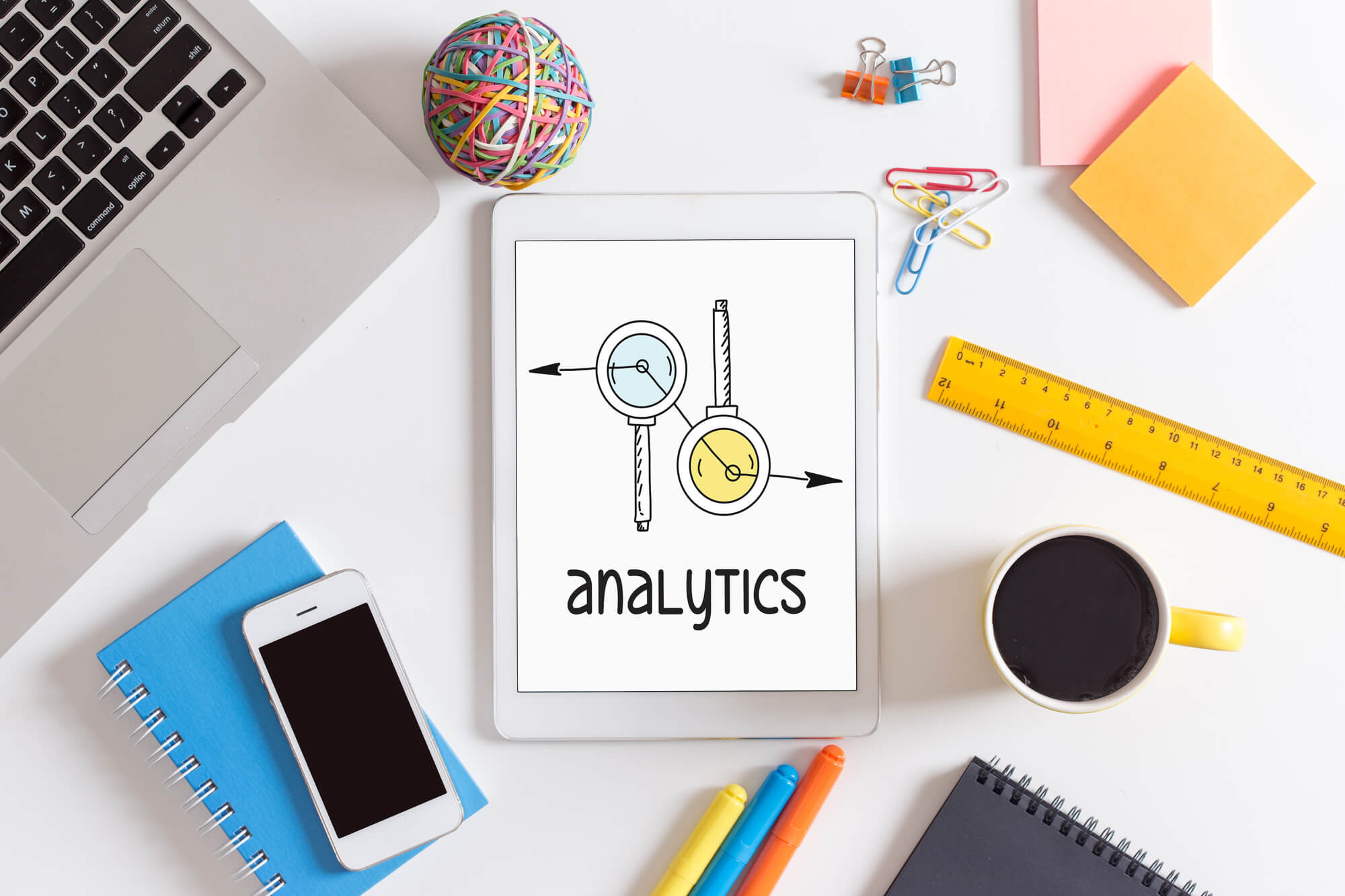
Equipment and equipment maintenance represent significant outlays of money, time and labor hours, which is one of the reasons predictive analytics have become so popular for equipment maintenance. Bringing technology to bear on the three classes of maintenance can greatly reduce the labor hours your business requires and help ensure greater uptime for your critical infrastructure. Here’s a look at how.
The three types of maintenance
When we talk about equipment maintenance, we’re usually talking about one of the following three types: condition-based maintenance (CBM), preventive maintenance (PM), and corrective maintenance (CM).
Today, we’re most interested in condition-based maintenance. In fact, in some circles, it’s even referred to as “predictive maintenance.” Thankfully, new technologies have taken a lot of the “predicting” out of “predictive maintenance.”
What is condition-based maintenance, and how do predictive analytics help?
Simply put, conditional maintenance is any work done on a piece of equipment when a need for service is recognized. It is work done after a certain conditional threshold is met, including after a part fails—or in this case, when it’s about to.
That’s the beauty of predictive analytics and where the business advantages of employing predictive models for condition-based maintenance really begin to shine. Consider the disruption of having a critical milling or stamping machine fail during a time of heavy demand. Think about all the machines you use to handle raw product or move merchandise from one point to another. All of these applications require a smooth operation and little to no downtime for a company to remain productive and profitable.
Predictive analytics can be used to study data gathered in real-time about the current operational performance of a piece of machinery. In a process broadly referred to as “condition monitoring,” any number of onsite monitoring devices—including telematic sensors and moisture and temperature probes—can be used to gather information about your infrastructure and the environment it’s operating in.
The ultimate goal of this network of connected machines and sensing equipment is to better anticipate and prioritize maintenance needs—meaning keeping downtime and engineer interventions to a minimum.
How does predictive analytics work?
Predictive analytics can be applied to any number of engineering problems, including the goal of reducing time spent performing maintenance, cutting machine downtime and ultimately keeping workflows efficient and uninterrupted.
When applied to the problem of designing more durable valves, for instance, analytical models can help engineers study hydrodynamic torque and other critical data points in a controlled, duplicable environment. This capability lets engineering and design teams spin up working prototypes faster and ultimately improve the product development process—another boon for many types of businesses.
Granted, using analytical and predictive models to improve equipment maintenance is a little different than using models to simulate product performance, even if the underlying principles are similar.
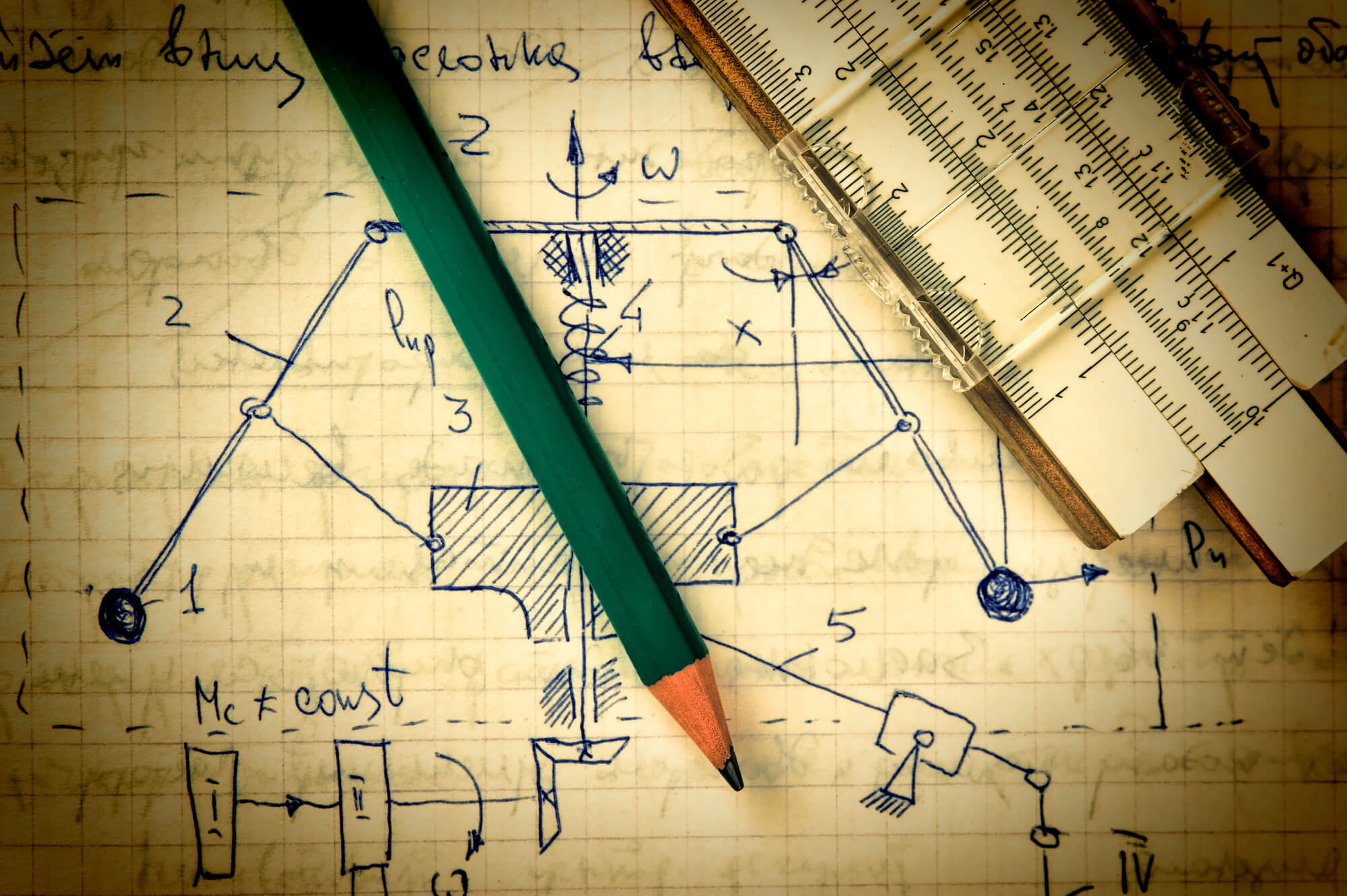
Predictive analytics can help engineers as they solve a myriad of problems such as cutting machine downtime. (Photo by DepositPhotos)
Here are some practical steps where predictive analytics and connected equipment can help improve your maintenance efforts and make life easier for engineers:
- Sensors enabled for telematics: These sensors can create models that help personnel better plan for equipment wear and degradation cycles. Plotting them over time lets business owners incorporate equipment maintenance cycles while drawing up employee schedules.
- Trend analysis and pattern recognition tools: Such tools can help equipment operators plot machine performance over time. This process helps pinpoint causal relationships between events and machine performance and lifetime. It can also reveal whether equipment is performing as it should or wearing down at the anticipated rate.
- Predictive analytics: Predictive analytics can be used when a purchasing manager or facility owner is weighing whether to repair or replace a piece of critical machinery that’s down for maintenance. Models that use relevant, real-world data gathered from equipment can more accurately plot a “remaining asset life” and plan future investments more accurately.
There are many reasons the manufacturing industry stands to benefit from more reliable analytical models. So far, our focus has been on maintaining the in-house infrastructure these entities require to keep production output high. But the same technologies can just as easily be applied to testing products themselves prior to them reaching the market.
As we mentioned above, stress-testing valve assemblies are one expected application for predictive analytics applied to product testing. For example, scale models in controlled testing environments can help designers and engineers reconcile conflicts between aesthetic product design and engineering requirements and determine the maximum and minimum operating conditions. The cost to recall a manufactured product can reach as high as hundreds of millions of dollars, including collecting faulty items from customers and paying applicable fines.
Granted, the analytical models you’re running are only as good as the software (or software vendor) you use to perform it. The right platform for your business or industry could be a toolset that ties together your industrial internet-of-things, aids with anticipating equipment maintenance, offers predictive modeling for product development and even models consumer demand over time. The benefits and opportunities of applying data-driven modeling are almost too numerous to name here.
Consider the alternative
The alternative to predictive maintenance and testing in manufacturing is reactive maintenance and testing. But running a critical machine to the point of failure isn’t good for the machine, and it doesn’t rely on a stable, predictable workflow for your organization.
No matter which way you cut it, you’re sabotaging yourself by not planning ahead. Building your maintenance cycles around predictive analytics is the much more logical approach when time and the wise use of resources are of the essence. And when aren’t they?
(Featured photo by DepositPhotos)
—
DISCLAIMER: This article expresses my own ideas and opinions. Any information I have shared are from sources that I believe to be reliable and accurate. I did not receive any financial compensation for writing this post, nor do I own any shares in any company I’ve mentioned. I encourage any reader to do their own diligent research first before making any investment decisions.

-
Biotech2 weeks ago
Spain Approves Groundbreaking Genetic ALS Treatment Tofersen for Public Use
-
Africa7 days ago
AfDB Gathers in Abidjan Amid Leadership Transition and Strategic Refocus
-
Crowdfunding2 weeks ago
Crowdfunding in France: From Boom to Slowdown and a Glimpse of Rebound
-
Business5 days ago
The TopRanked.io Weekly Digest: What’s Hot in Affiliate Marketing [Masters of Trivia Referral Program]
You must be logged in to post a comment Login